Introduction to Deep Learning
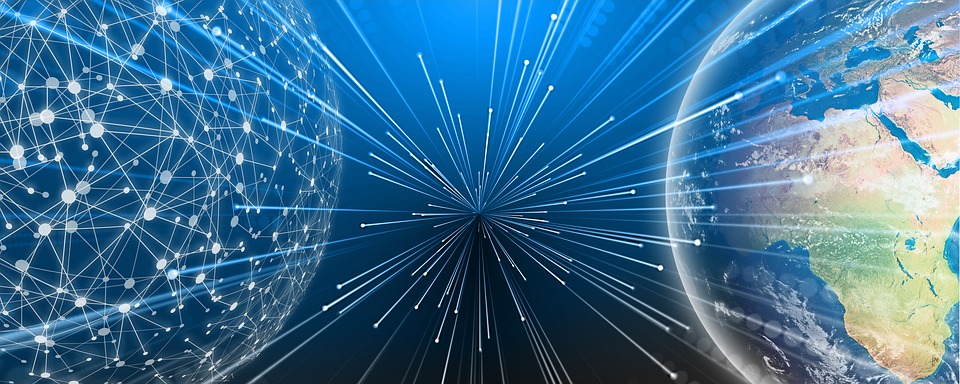
ABOUT THE COURSE!
Deep learning can be considered as a subset of machine learning. It is a field that is based on learning and improving on its own by examining computer algorithms. While machine learning uses simpler concepts, deep learning works with deep neural networks, which are designed to imitate how humans think and learn.
The fourth course of the Data Science Program aims at providing you a fundamental of modern neural networks and their various applications on computer vision and natural language processing. Furthermore, learners will be able to build Deep Neutral Networks models with two of the most popular deep learning library – Tensorflow and Keras provided by Google. Various optimization techniques also have been taught for fine-tuning a DNN model.
To begin the course, let's take a few minutes to explore the course site. Review the material we’ll cover each week, and preview the assignments/projects/quizzes you’ll need to complete to pass the course.
Main concepts are delivered through videos, demos and hands-on exercises.
COURSE INFORMATION
Course code: | DSP304x |
Course name: | Introduction to Deep Learning |
Credits: | 3 |
Estimated Time: | 6 weeks. Student should allocate at average of 2 hours/ day to complete the course. |
COURSE OBJECTIVES
After taking this course, the students should all be able to:- Comprehend about gradient descent, stochastic gradient descent, regularization, overfitting
- Acquire overview and basic knowledge about deep neural network
- Introducing Deep Learning in Computer Vision. Be able to apply CNN and transfer learning to computer vision tasks.
- Acquire overview and basic knowledge about unsupervised representation learning in deep learning as autoencoder, word embedding.
- Introducing Deep Learning in NLP/sequence models. Be able to apply RNN, LSTM to GRU to NLP tasks.
- Proficiently manipulate typical and basic libraries in machine learning with Python: Numpy, Tensorflow, Keras.
COURSE STRUCTURE
Module 1 - Simple Neutral Network
- Lesson 1: Introduction to Optimization
- Lesson 2: Stochastic methods for optimization
- Lesson 3: Introduction to Neutral Network
Module 2 - Deep Learning in Computer Vision
- Lesson 4: Deep Learning Framework
- Lesson 5: Deep Learning for Images
- Lesson 6: Applications of CNNs
Assignment 1 - Project - Image Classification
Module 3 - Unsupervised Representation Learning
- Lesson 7: Unsupervised Learning
Module 4 - Deep Learning in Natural Language Processing
- Lesson 8: Word Embedding
- Lesson 9: Introduction to RNN
- Lesson 10: Modern RNNs
Assignment 2 - Project - Toxic comment classification
DEVELOPMENT TEAM
COURSE DESIGNERS
M.Sc. Nguyen Hai Nam
|
M.S. Vu Thuong Huyen
|
REVIEWERS & TESTER
Course Reviewer
|
Course Tester
|
||
Ph.D. Dang Hoang Vu |
|
B.A. Ho Quoc Bao |
|
Assoc. Prof. Tu Minh Phuong |
Ph.D. Nguyen Van Vinh |
Ph.D. Tran The Trung |
|
|
|
MOOC MATERIALS
Below is the list of all free massive open online learning sources (MOOC) from Coursera used for this course by FUNiX:
Learning resources
In modern times, each subject has numerous relevant studying materials including printed and online books. FUNiX Way does not provide a specific learning resource but offers recommendation for students to choose the most appropriate source to them. In the process of studying from many different sources based on that personal choice, students will be timely connected to a mentor to respond to their questions. All the assessments including multiple choice questions, exercises, projects and oral exams are designed, developed and conducted by FUNiX.
Learners are under no obligation to choose a fixed learning material. They are encouraged to actively find and study from any appropriate sources including printed textbooks, MOOCs or websites. Students are on their own responsibilities in using these learning sources and ensuring full compliance with the source owners’ policies; except for the case in which they have an official cooperation with FUNiX. For further support, feel free to contact FUNiX Academic Department for detailed instructions.
Learning resources are recommended below. It should be noted that listing these learning sources does not necessarily imply that FUNiX has an official partnership with the source’s owner: Coursera, tutorialspoint, edX Training, Udemy, Machine Learning cơ bản, or Towards Data Science.
Feedback channel
FUNiX is ready to receive and discuss all comments and feedback related to learning materials via email program@funix.edu.vn